Understanding the Significance of **Data Labeling Platforms** in Modern Business
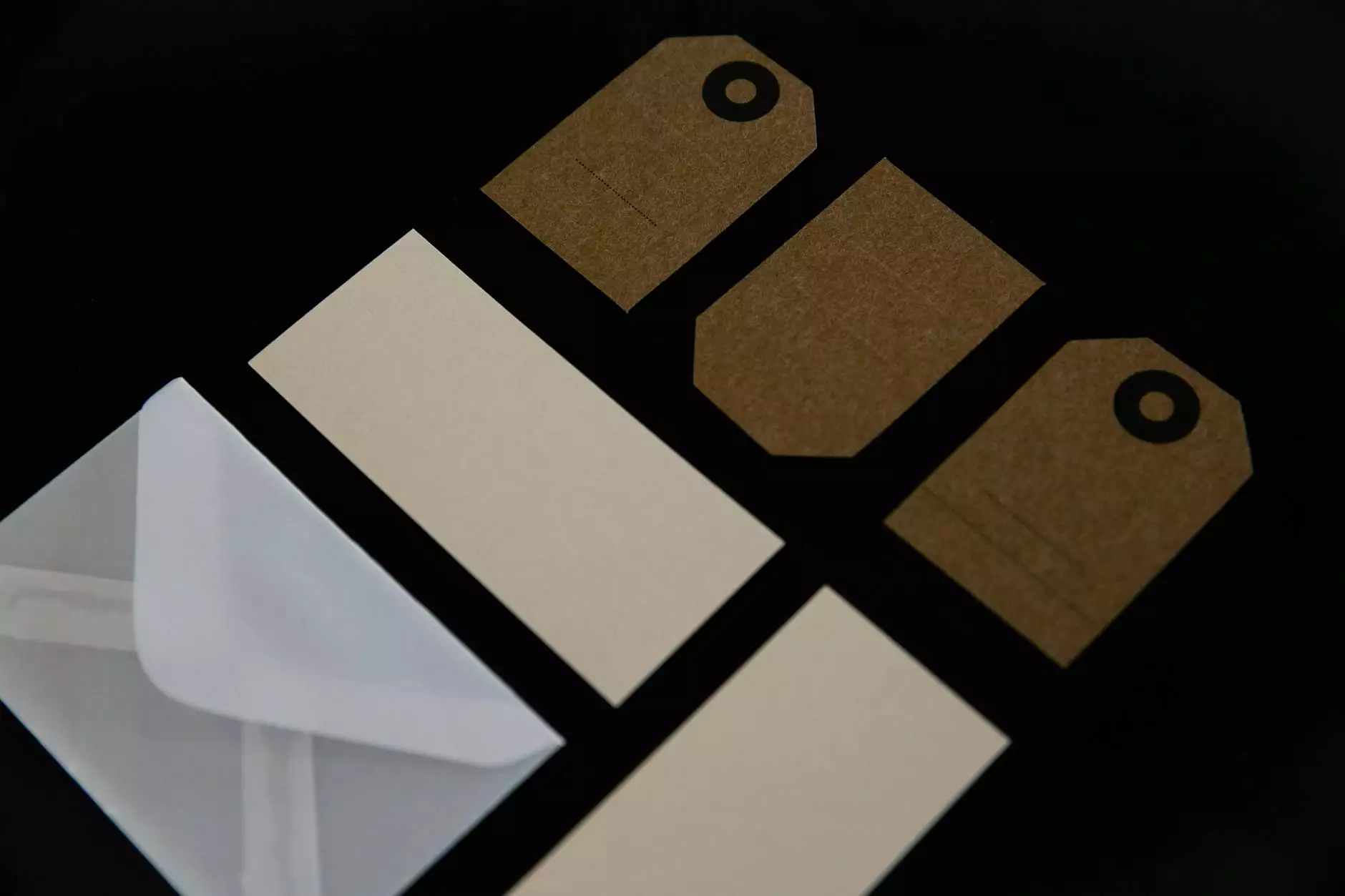
In the digital age, businesses across various sectors are increasingly relying on data-driven decisions to stay competitive. One crucial element in this data-centric world is the role of data labeling platforms. These platforms are indispensable tools that enhance the efficiency and accuracy of machine learning (ML) and artificial intelligence (AI) applications. This article delves deep into the functionalities, benefits, and the future landscape of data labeling, ensuring you understand why it is pivotal for businesses today.
What is a Data Labeling Platform?
A data labeling platform is a specialized tool or software that facilitates the process of annotating datasets. Annotation involves the categorization and identification of data attributes to provide context and meaning. This process is essential for training machine learning models to make accurate predictions or classifications.
Key Features of Data Labeling Platforms
- Scalability: Many platforms offer scalable solutions that can handle varying volumes of data, from small projects to large-scale enterprise needs.
- Collaboration Tools: They enable teams to work together seamlessly, ensuring that data is labeled consistently across views and perspectives.
- Quality Assurance: Most data labeling platforms implement quality checks to ensure that the labels provided are accurate, thereby improving the overall quality of the ML models.
- Integration Capabilities: They often integrate easily with other tools in the data science ecosystem, making preprocessing and labeling a smooth process.
The Role of Data Labeling in Machine Learning
Machine learning algorithms require labeled data to "learn" from. For example, image recognition systems need labeled images to differentiate between various objects. This is where data labeling platforms come into play. They provide the necessary infrastructure to annotate large datasets required for effective ML training.
Types of Data Labeling
Data labeling can take many forms. Here are some common types:
- Image Annotation: Used in computer vision, this involves drawing bounding boxes, segmentations, and key points around objects in images.
- Text Annotation: This method involves tagging words or phrases in text data to help sentiment analysis, entity recognition, etc.
- Audio Annotation: Involves transcribing audio clips and labeling parts of the audio data for applications like speech recognition.
- Video Annotation: Similar to image annotation but applied to video sequences, often used for object tracking and activity recognition.
Benefits of Using Data Labeling Platforms
The incorporation of data labeling platforms in business processes can yield numerous benefits:
1. Enhanced Accuracy and Quality
High-quality labeling is critical for the success of any machine learning project. Platforms often utilize expert labelers, thus increasing the accuracy of the labeled data. This, in turn, enhances the performance of machine learning models, leading to better business insights.
2. Cost Efficiency
Data labeling can be labor-intensive, but outsourcing this task to a dedicated platform can prove to be more cost-effective than managing in-house teams. Businesses can save on recruitment, training, and infrastructure costs.
3. Speed and Volume
Efficient labeling helps businesses process larger datasets in shorter time frames. This rapid data acquisition allows companies to stay ahead of competitors by making timely data-driven decisions.
4. Focus on Core Competencies
By utilizing data labeling platforms, businesses can allocate their CI resources towards core competencies instead of spending valuable time on data preparation tasks.
How to Choose the Right Data Labeling Platform?
Choosing the right platform can make a significant difference in your data projects. Here are some factors to consider:
1. Understand Your Data Needs
Assess the type and volume of data you need to label. Different platforms specialize in different kinds of data annotations such as images, text, or audio.
2. Evaluate Quality Assurance Measures
Look for platforms that offer quality checks and a feedback mechanism to ensure the accuracy of the annotations.
3. Consider Budget and Cost Structures
Different platforms have various pricing models. Make sure to understand your options—whether they charge per hour, per labeled item, or a subscription fee.
4. Integration with Current Workflows
Your chosen platform should integrate seamlessly with your existing data pipelines and software tools to ensure a smooth workflow.
Case Studies: Successful Implementation of Data Labeling Platforms
1. Healthcare
The healthcare industry has leveraged data labeling platforms to improve diagnostics using image recognition. By annotating thousands of medical images for training algorithms, healthcare organizations have been able to enhance their diagnostic capabilities significantly.
2. Autonomous Vehicles
In the realm of self-driving cars, data labeling is essential. Platforms are used to annotate the vast amount of video and image data collected by vehicle cameras to recognize obstacles, lanes, and pedestrians, ensuring safer autonomous driving systems.
3. E-commerce
E-commerce businesses employ data labeling to analyze customer sentiments and enhance recommendation systems. By tagging customer reviews and feedback, these platforms help in building more effective personalized marketing strategies.
The Future of Data Labeling Platforms
The future of data labeling platforms looks promising. With advancements in AI and ML, automated data labeling tools are on the rise. While human intelligence will still play a critical role in complex tasks, AI-assisted labeling can significantly speed up the process and improve cost-efficiency.
Trends to Watch
- Increased Use of Automation: As AI improves, automation in data labeling processes will become more prevalent, resulting in faster turnaround times.
- Multi-Modal Data Support: Future platforms will likely support multi-modal data types, combining text, image, audio, and video to create richer datasets.
- Real-Time Collaboration: Enhanced collaborative tools will revolutionize how teams work together on data labeling projects.
- Ethical Considerations: As data privacy concerns grow, platforms will need to adopt best practices to ensure compliance and ethical handling of data.
Conclusion
In an era where data drives decision-making, the importance of effective data labeling cannot be overstated. Data labeling platforms serve as an essential bridge between raw data and actionable insights, enabling businesses to harness the full power of their data for machine learning applications. By understanding the complexities and benefits of these platforms, businesses can position themselves to thrive in a data-driven world.
As industries continue to evolve, the role of data labeling is set to expand. Embracing these tools will not only optimize your data processing but will also lead to innovative solutions and a competitive edge in your market.
Investing in data labeling platforms is, therefore, not just a decision for efficiency—it’s a strategic move towards ensuring sustained growth and success in your business.